Delve into the realm of statistics with the AP Statistics Chapter 10 Test Answer Key PDF, your comprehensive guide to unlocking the mysteries of sampling distributions and hypothesis testing. This invaluable resource empowers you to conquer the intricacies of statistical inference, providing a solid foundation for success in AP Statistics and beyond.
Prepare to embark on an enlightening journey as we explore the fundamental concepts of sampling distributions, unravel the significance of the Central Limit Theorem, and delve into the practical applications of hypothesis testing and confidence intervals. With this key in hand, you’ll gain a deeper understanding of statistical reasoning and its indispensable role in scientific research and decision-making.
Chapter 10: Sampling Distributions and Hypothesis Testing
Chapter 10 of AP Statistics introduces the concept of sampling distributions and their role in hypothesis testing and confidence intervals. This chapter is crucial for understanding how statisticians make inferences about populations based on sample data.
1. Overview of Chapter 10 in AP Statistics
In this section, we will define the concept of sampling distributions, explain the Central Limit Theorem and its significance, and discuss the different types of sampling distributions.
Sampling Distributions: A sampling distribution is a probability distribution of a statistic calculated from all possible samples of a given size from a population.
Central Limit Theorem: The Central Limit Theorem states that the sampling distribution of the sample mean will be approximately normal, regardless of the shape of the population distribution, as long as the sample size is large enough (n > 30).
Types of Sampling Distributions: There are several types of sampling distributions, including the sampling distribution of the sample mean, the sampling distribution of the sample proportion, and the sampling distribution of the sample standard deviation.
2. Hypothesis Testing Using Sampling Distributions
In this section, we will describe the null and alternative hypotheses, explain the steps involved in hypothesis testing, and discuss the role of p-values in hypothesis testing.
Null Hypothesis: The null hypothesis (H0) is a statement that there is no significant difference or relationship between the variables being tested.
Alternative Hypothesis: The alternative hypothesis (Ha) is a statement that there is a significant difference or relationship between the variables being tested.
Steps in Hypothesis Testing: The steps involved in hypothesis testing include stating the hypotheses, setting the significance level, calculating the test statistic, determining the p-value, and making a decision.
P-Values: A p-value is the probability of obtaining a test statistic as extreme as or more extreme than the one observed, assuming the null hypothesis is true.
3. Confidence Intervals for Proportions
In this section, we will define a confidence interval and its components, explain how to calculate a confidence interval for a proportion, and discuss the factors that affect the width of a confidence interval.
Confidence Interval: A confidence interval is a range of values that is likely to contain the true population parameter with a certain level of confidence.
Components of a Confidence Interval: A confidence interval consists of a lower bound, an upper bound, and a confidence level.
Calculating a Confidence Interval for a Proportion: To calculate a confidence interval for a proportion, we use the formula: “` sample proportion +/- margin of error “` where the margin of error is: “` z – sqrt((sample proportion – (1 – sample proportion)) / sample size) “`
Factors Affecting the Width of a Confidence Interval: The width of a confidence interval is affected by the sample size, the sample proportion, and the confidence level.
4. Applications of Hypothesis Testing and Confidence Intervals, Ap statistics chapter 10 test answer key pdf
In this section, we will provide real-world examples of how hypothesis testing and confidence intervals are used, explain how to interpret the results of hypothesis tests and confidence intervals, and discuss the limitations of hypothesis testing and confidence intervals.
Real-World Examples: Hypothesis testing and confidence intervals are used in a wide variety of fields, including medicine, psychology, and business.
Interpreting the Results: The results of hypothesis tests and confidence intervals can be interpreted in terms of statistical significance and practical significance.
Limitations: Hypothesis testing and confidence intervals have certain limitations, such as the possibility of Type I and Type II errors.
Q&A: Ap Statistics Chapter 10 Test Answer Key Pdf
What is the significance of the Central Limit Theorem?
The Central Limit Theorem establishes that regardless of the shape of the population distribution, the sampling distribution of means will approach a normal distribution as the sample size increases. This remarkable property allows us to make inferences about population parameters based on sample data.
How do I calculate a confidence interval for a proportion?
To calculate a confidence interval for a proportion, use the formula: sample proportion ± margin of error. The margin of error is determined by the sample size, confidence level, and standard deviation of the population.
What are the limitations of hypothesis testing?
Hypothesis testing is a powerful tool, but it has certain limitations. It can only provide evidence against the null hypothesis, not prove it. Additionally, hypothesis testing is sensitive to sample size, and a larger sample size may lead to rejection of the null hypothesis even when the effect size is small.
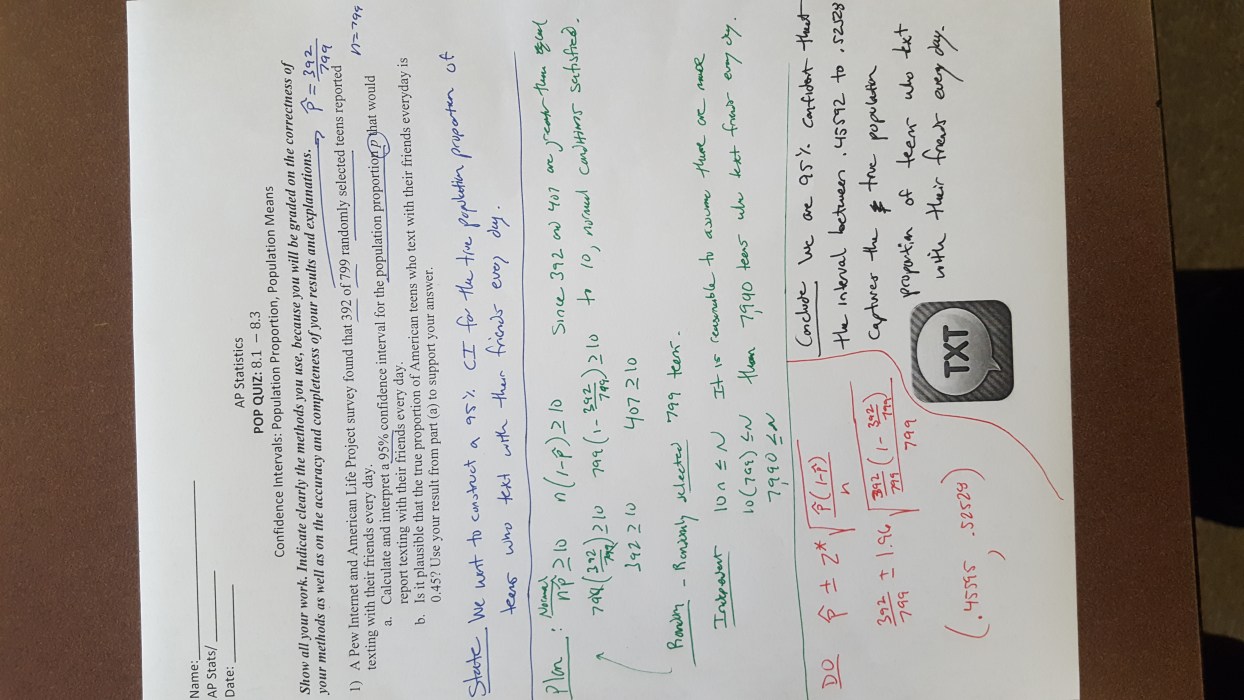